Jens Ammon
Geostatistics with Sasquatch
In this exercise we explore geospatial statistics tools to examine the locational patterns, auto-correlation values, and statistically significant clusters of Bigfoot activity in the United States. The primary questions we are looking to answer are: 1. Do Bigfoot sightings occur randomly across the US? and 2. Are sightings evenly dispersed across the landscape or are they more common in certain areas? To answer these questions, spatial groupings are made using both the location of the data and attributes of sighting report reliability.

The map to the left shows the distribution of Sasquatch sightings across the United States. The hot spot distribution is based on location density of sightings and the quality or reliability of those sighting reports. The distance band, or radius around each point used when analyzing neighbors, is fixed at 50 km.
​
Areas in red indicate a high density of reliable sightings. Areas in blue indicate a cluster of poor reliable sightings. Sighting source reliability does not necessary mean it will will be near sightings of similar reliability, unless there are actually bigfoot in the area.
Average Nearest Neighbor Analysis
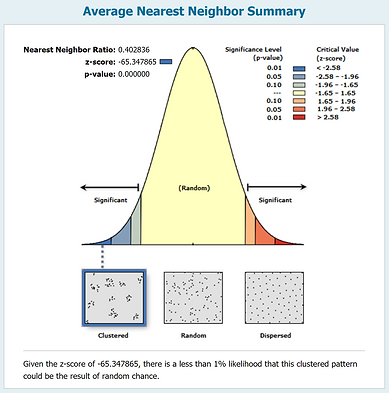

The Average Nearest Neighbor tools gives a nearest neighbor index value based on how the mean distance of the dataset compares to the "expected" or theoretical mean distance if the data were truly randomly distributed. This index value, or Nearest Neighbor Ratio, then relates to whether the data is clustered (ratio < 1) or disperse (ratio >1). This analysis is based purely on the location of the data points and does not account for any other attributes that may possibly link points together.
​
If the sightings were randomly distributed across the country (the dataset area), the average distance between sightings would be equal to the Expected Mean Distance. In the table above, the Observed Mean Distance is what this average distance between sightings actually is for the dataset. Since the Observed Mean Distance (19064 m) is less than the Expected Mean Distance (47325 m), more points are closer together than what would be in a random distribution. The Nearest Neighbor Ratio is a singular number to represent this difference between distances (Observed Mean Distance / Expected Mean Distance), and since the ratio is less than 1, it indicates the bigfoot sighting data is clustered.
​
The P-value is a ranking of the probability of the data being created from random occurrence. Since the P-value is low, it is likely the sightings did not occur randomly.
Moran's I Analysis

The Global Moran's I tool gives a correlation of the data based on both the geospatial location of the points and an attribute. In this scenario, the attribute used to determine was a ranked scale of the reliability of the sighting report. Similar to the Nearest Neighbor Analysis, the Moran's I analyzes the overall dataset and tells whether the data is Dispersed, Random, or Clustered. The Moran's Index ranges from negative to positive values, with I < 1 indicating a dispersed distribution, I = 0 indicating a random distribution, and I > 1 indicating a clustered distribution. Coupled with the P-value results, the Moran's Index can suggest the presence or absence of a pattern too strong for random chance.
​
With the Moran's Index of 0.179 and the P-value of 0 for this dataset, it is very likely the sasquatch sightings are occurring in a clustered pattern instead of appearing by random chance. The high z-score, or amount of standard deviations away from the mean, also supports the conclusion that the sightings are clustered in a statistically significant way.

Hot Spot Analysis (above map)
The Hot Spot Analysis (Getis-Ord Gi*) tool differs from the Nearest Neighbor and Moran's I tools in that the Hot Spot tool analyzes local clusterings of data points. This analysis does not say whether the data distribution is clustered or random. Rather, it visualizes where local groupings occur. That is, it shows where the high values are that also have high-value neighbors (or low value data points that also have low-value neighbors). Like the Moran's I analysis, the Hot Spot analysis can be weighted based on a particular attribute. With this attribute, data density can be normalized, such as by areas where the data values are expected to be artificially higher - e.g. more house foreclosures in areas with more homes, or more bigfoot sightings in areas with more visitors.
​
For the above map, the radius used to analyze neighboring points was manually fixed. The resulting groupings of hot and cold spots relies heavily on the radius chosen in the analysis. In order to define particular regions of sasquatch likelihood, a small radius of 50 km was used relative to the extent of the dataset.